【開催日:4/28(月)】電気学会中国支部講演会(主催)
2025/04/17
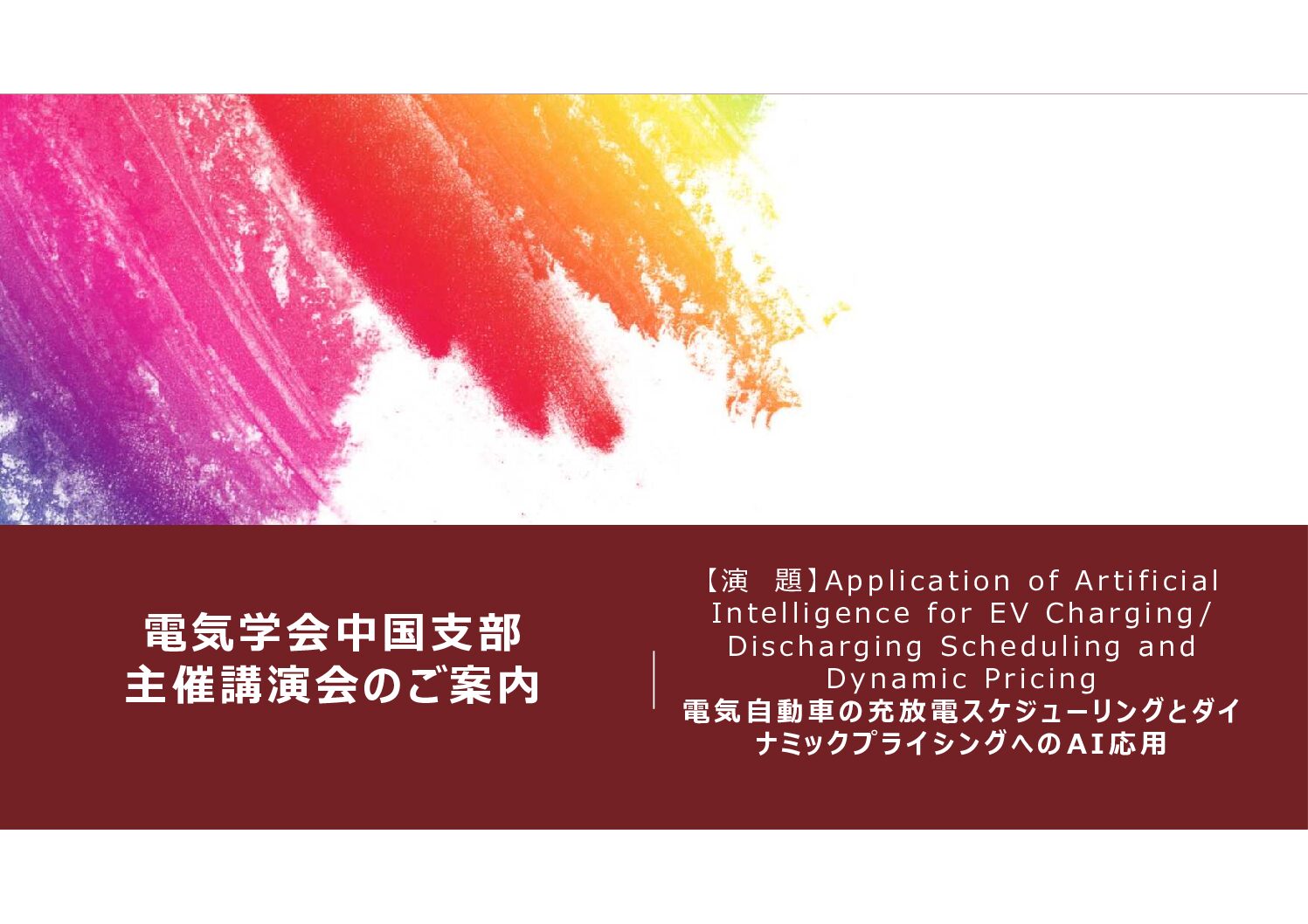
講 演 会 の ご 案 内
【日 時】2025年4月28日(月) 15:00~16:00
【場 所】広島大学(東広島キャンパス)工学部A1棟1階141会議室(〒739-8527東広島市鏡山1-4-1)
【演 題】Application of Artificial Intelligence for EV Charging/ Discharging Scheduling and Dynamic Pricing
電気自動車の充放電スケジューリングとダイナミックプライシングへのAI応用
【概 要】
The number of EVs will continue to grow with governments’ incentives and policies around the globe. On the one hand, the increasing and uncoordinated EV charging will burden the existing power grid. On the other hand, EVs’ batteries are mobile energy storage systems that can be used to provide ancillary services for power grids, such as peak-shaving and valley- filling, voltage and frequency regulations. In addition, EVs’ batteries can be used as flexible load and supply to maximize renewable energy utilization, if EVs charging and discharging are properly coordinated to closely match renewable generation profiles. Using EVs as energy storage through the V2G program can bring many environmental, economic, and social- technological benefits to program participants, such as EV owners, grid operators, government, and aggregators. Many EV charging scheduling approaches have been effective in following Time of Use (ToU) and Real-Time Pricing (RTP) signals for peak demand reduction, alleviating the impact of load fluctuation, and reducing EV charging costs. However, the effectiveness of these pricing policies in reflecting power system conditions has barely been discussed in the literature. Dynamic pricing is a special form of demand response that can encourage EV owners to participate in scheduling programs. Therefore, EV charging and discharging scheduling and its dynamic pricing model are important fields of study. Many researchers have focused on artificial intelligence-based EV charging demand forecasting and scheduling models and suggested that artificial intelligence techniques perform better than conventional optimization methods. However, only a few research studies focused on EV discharging scheduling (i.e., vehicle-to-grid-V2G) because the concept of discharging electricity back to the power grid by EV is relatively new and evolving. Therefore, a review of existing EV charging and discharging-related studies is needed to understand the research gaps and to make some improvements in future studies. In this talk, I will review EV charging/discharging- related studies and classify them into forecasting, scheduling, and pricing mechanisms. I will compare, summarize and analyze the existing artificial intelligence-based algorithms of the three critical EV charging/discharging components: forecasting, scheduling, and dynamic pricing. I will then discuss an example where multi-agent reinforcement learning is used for dynamic pricing for EV Charging/Discharging.
各国政府の奨励策や政策により、電気自動車の数は今後も増加していくことが予想される。 一方、無秩序に増加する電気自動車の充電は、既存の電力網に負担をかけることにもつながる。電気自動車(EV)のバッテリーは移動式エネルギー貯蔵システムであり、電力需要のピークカットなど、電圧や周波数の調整など、電力ネットワークにおける補助的なサービスとしても利用可能である。さらに、EVの充電と放電を適切に調整し、再生可能エネルギーの発電プロファイルにできるだけ近づけることができれば、EVのバッテリーを柔軟な負荷および供給源として活用し、再生可能エネルギーの利用を最大限に高めることも可能である。V2Gプログラムを通じてEVをエネルギー貯蔵として利用することで、EVの所有者、送電網の運営者、政府、アグリゲーターなど、プログラムの参加者に環境面、経済面、社会技術面での多くのメリットをもたらすことができる。多くのEV充電スケジュール手法は、ピーク需要の削減、負荷変動の影響の緩和、EV充電コストの削減を目的とした時間帯別料金(ToU)やリアルタイム料金(RTP)のシグナルに効果的に対応している。しかし、電力系統の状態を反映するこれらの料金政策の有効性については、ほとんど議論がなされていない。ダイナミックプライシングは、EV所有者にスケジュールプログラムへの参加を促すことができる特別なデマンドレスポンスの形態である。したがって、EVの充電と放電のスケジュールと、そのダイナミックプライシングモデルは重要な研究分野である。多くの研究者は、人工知能に基づくEV充電需要予測とスケジュールモデルに焦点を当て、人工知能技術が従来の最適化手法よりも優れていることを示唆している。しかし、EVによる電力網への放電という概念は比較的新しく、進化しているため、EV放電スケジュール(V2G)に焦点を当てた研究はわずかである。したがって、研究上のギャップを理解し、今後の研究に改善を加えるためには、既存のEV充電および放電関連の研究をレビューする必要がある。本講演では、EV充電/放電関連の研究をレビューし、予測、スケジューリング、価格メカニズムに分類する。EVの充電/放電の3つの重要な要素である予測、スケジューリング、動的価格設定について、既存のAIベースのアルゴリズムを比較、要約、分析する。次に、EVの充電/放電の動的価格設定にマルチエージェント強化学習が使用された例について説明する。
【講 師】Prof. Komla Folly (University of Cape Town, South Africa)
【問合せ先】広島大学 大学院先進理工系科学研究科 電力・エネルギー工学研究室
造賀 芳文,佐々木 豊
Tel:082-424-7668(佐々木直通),Fax:082-424-3586
e-mail:yusasaki@hiroshima-u.ac.jp(佐々木)
【参加費】 無 料
【事前申込】不 要
【主 催】 電気学会中国支部
【共 催】募集中
以 上